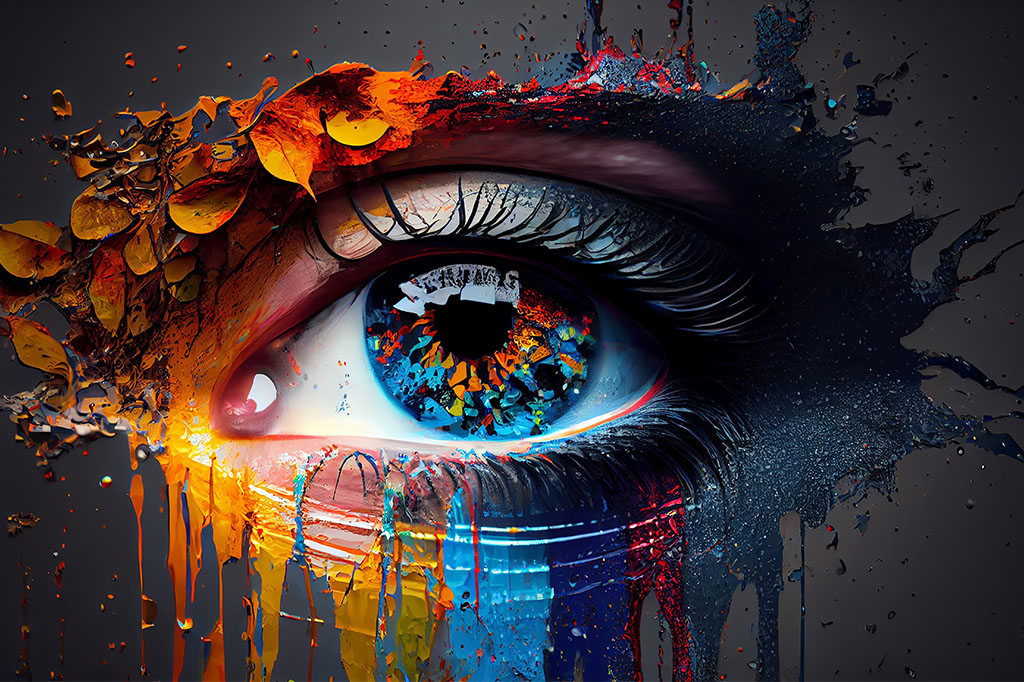
Generative Artificial Intelligence (GAI) has revolutionized the way we understand content creation and decision-making from data. Systems like ChatGPT or Dall-E possess a unique ability to produce new and original results based on what they’ve learned.
What is Generative Artificial Intelligence?
Generative Artificial Intelligence refers to AI systems that can create or generate something new from previously learned data. Instead of simply analyzing or processing information, these systems can “invent” or “devise” content, solutions, or concepts that didn’t previously exist in the data they were trained with.
How Does Generative Artificial Intelligence Work?
Deep Learning Models: Most GAI solutions rely on deep neural networks (DNNs) capable of identifying complex patterns in large data sets. These networks are “trained” with substantial data amounts and adjusted to generate original outputs based on this data.
Generative Techniques: Methods like Generative Adversarial Networks (GANs) are a prominent example of GAI. In a GAN, two neural networks (the generator and the discriminator) work together. While the generator tries to create data, the discriminator attempts to distinguish between real data and data generated by the generator. Through iterations, the generator improves.
Specific Data Structures: Depending on what you want to generate (text, images, music), different data structures and network architectures are used. For text, recurrent neural networks (RNNs) or Transformers might be particularly effective, while convolutional networks (CNNs) are often the preferred choice for images.
Optimization and Fine-Tuning: Once the basic model is developed, various optimizations and fine-tunings are performed to improve its ability to generate high-quality and diverse results. This might include adjusting hyperparameters, incorporating additional data, or modifying the model’s architecture.
Music Generation
In the field of Autonomous Composition, there are several notable applications revolutionizing music creation.Sony’s Flow Machines is an AI tool that collaborates with human artists to generate music in various styles. Similarly, IBM Watson Beat uses deep learning to assist artists in creating original compositions based on user-provided audio samples. On the other hand, Aiva specializes in classical melodies, producing scores performed by professional orchestras later. Other platforms like Amper Music, designed by film composers, facilitate swift background music creation with a focus more on musical theory than neural networks. Google’s project Magenta explores the AI limits in the process of creating art and music. Jukedeck, a British startup, creates soundtracks using AI, and Humtap transforms simple humming or voices into original soundtracks.
For Accompaniment and Base Creation, DistroKid’s AI-driven VST is a valuable tool that provides musical accompaniment based on a melody introduced by the user.
For Improvement and Sound Mastering, LANDR has established itself as a leading platform using AI for automatic track mastering, allowing artists to achieve professional sound.
In Voice Generation, we are still exploring the vast possibilities AI can offer, with systems capable of simulating singing voices based on text or provided melodies.
Image Generation
The revolution of Artificial Intelligence in image generation and design has reached a climax in recent years. Tools like OpenAI’s Dalle-2, Bluewillow, Craiyon (formerly known as Dalle-mini), and Stability AI’s Dreamstudio, among others, allow both amateurs and professionals to create stunning visual works from simple descriptions, sketches, or keywords.
Platforms like Midjourney stand out, offering an interface through Discord where users can obtain four variations of an image after entering a description. Another example is Nightcafe, which not only generates AI-based visualizations but also allows for the printing of creations, giving a new twist to the materialization of digital art.
In addition, Stability AI solutions, like Stable Diffusion and Stable Doodle, offer a range of options for generating images, either from texts or sketches, with results that are surprising for their accuracy and quality. In the case of Stable Doodle, the tool can interpret and enhance simple outlines of a drawing, offering artistic variations according to the selected style.
However, despite the wonders that these technologies offer, they are not exempt from ethical debates and controversies. The line between inspiration and technological plagiarism has become more blurred. Examples like Jason Allen and his award-winning work “Théâtre D’opéra Spatial” generated by MidJourney have sparked discussions about authenticity and the true meaning of art in this digitalized era. Divided opinions date back to the time when photography was considered a threat to traditional art.
Text Generation
AI is transforming text content generation. Tools like GPT-4 from OpenAI and BERT from Google have paved the way for a new era of assisted writing. GPT-4 impresses with coherent results on various topics, while BERT excels in context interpretation. BERT excels in context interpretation T5 redefines language problems as text-to-text translations, Salesforce’s CTRL produces text under specific conditions, and XLNet improves the coherence and naturalness of the generated text by predicting words in all positions.
The market also offers innovative solutions for specific needs. Jasper AI focuses on advertising content creation, while Rytr and Copy.AI offer SEO optimization. Hypotenuse focuses on integration with e-commerce platforms.
However, these technologies are not without ethical debates and controversies. The line between inspiration and technological plagiarism has blurred, sparking discussions about authenticity and art’s true meaning in this digitalized era.Divided opinions date back to when photography was considered a threat to traditional art.
Other Applications of Generative Artificial Intelligence
Beyond generating music, text, and images, GAI has the potential to create and edit videos, design games and 3D models, control robots performing complex tasks, simulate scenarios ranging from climate systems to biological interactions, and analyze patterns in large data sets. It can interpret human emotions to improve chatbots, translate audio and video in real-time, and design adaptive educational programs. In healthcare, GAI can be a crucial tool for diagnostic imaging or designing molecular structures for new drugs.
Generative Artificial Intelligence has emerged as a powerful catalyst in the innovation of multiple fields. Its ability to accelerate creative processes has been evident in industries such as film, music, and design. Additionally, it offers personalized solutions in critical sectors like medicine and architecture, adapting to specific needs. Moreover, its ability to explore and navigate through complex spaces surpasses human limitations, opening doors to domains previously inaccessible or difficult to approach.
However, GAI is not without challenges. The tension between originality and quality is palpable: genuinely new content is not always synonymous with high quality or relevance. In the realm of ethics and responsibility, questions arise about the authorship and direct responsibility for the creations generated by GAI, particularly in artistic and literary fields. Furthermore, if GAI is trained with data containing specific preferences, these viewpoints may be reflected in its results.